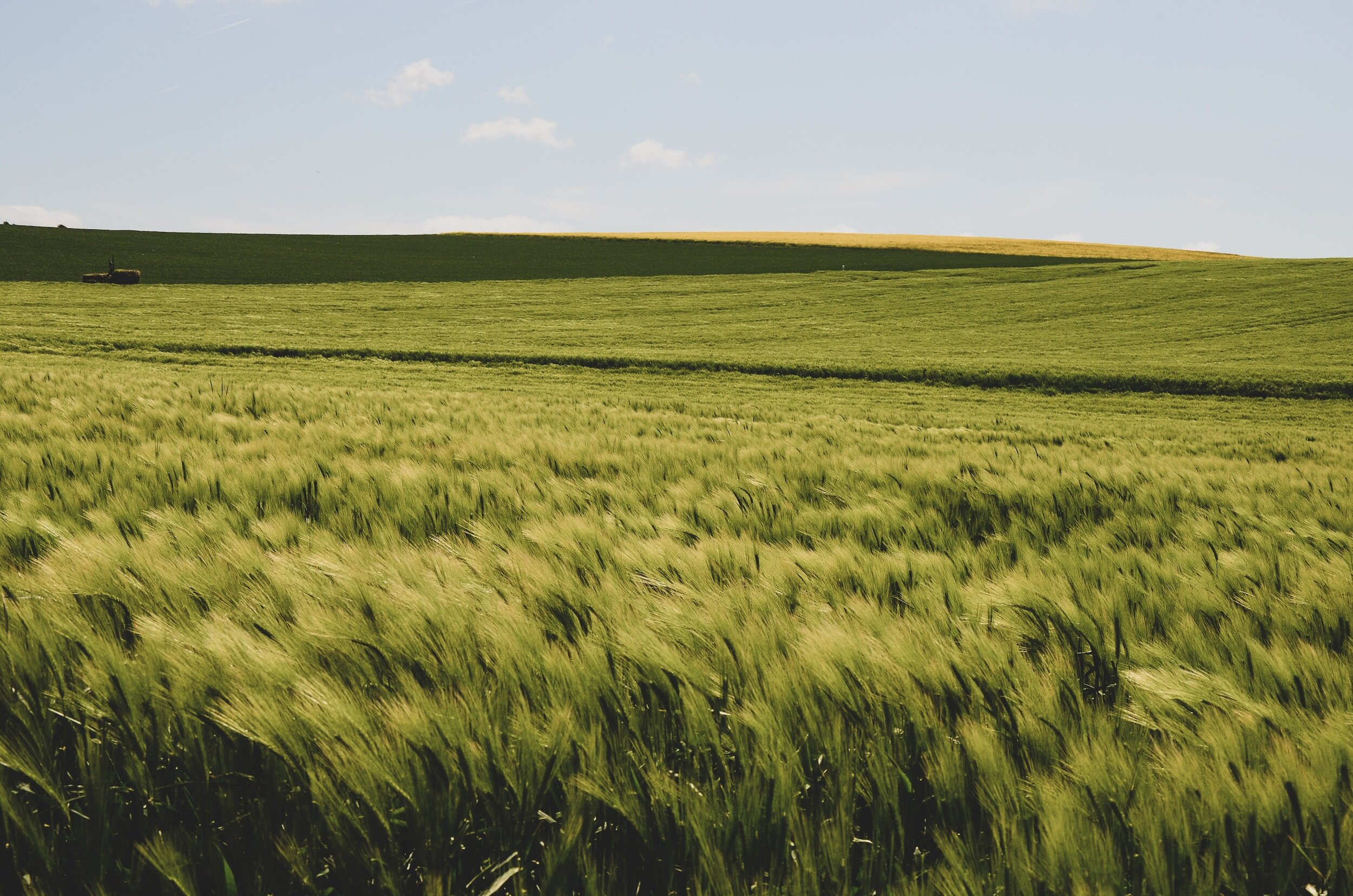
Predict, Prepare, Prosper:
Embrace the Revolution in Grain Production Analysis
Crop Yield Prediction
Client Background:
A prominent agricultural co-operative dedicated to supporting its farmers with a comprehensive range of products and services, including agronomy, grain, feed, farm supply, and energy. The co-operative aimed to optimize their grain yield forecasts to enhance hedging, streamline logistics, and align insurance strategies.
Objectives:
Refine grain yield forecasts for targeted grains.
Enhance the co-operative's hedging strategies based on accurate yield predictions.
Streamline logistics, including rail and freight, based on forecasted yields.
Align insurance strategies to better match anticipated grain yields.
Challenge:
The co-operative sought to refine its grain yield forecasts to make more informed decisions regarding hedging, logistics, and insurance, ultimately benefiting its farmer members and optimizing operations.
Approach:
Data Collection:
Traditional Data Sources: Our team gathered production data for corn, soybeans, and wheat across six major grain-producing states. We extracted data on acreage, prevent plant acres, yield, and production from major producing counties in each state.
Advanced Satellite Imagery: Leveraging cutting-edge satellite technology, we obtained high-resolution imagery to assess crop health, soil moisture, and vegetation indices, providing a more granular view of potential yields.
Remote Sensing Data: Our proprietary sensors deployed in select locations captured micro-climatic data, offering insights into local weather conditions that could impact grain growth.
IoT Integration: We integrated data from Internet of Things (IoT) devices placed in strategic locations, capturing real-time data on soil health, moisture levels, and other critical parameters.
Proprietary Algorithms: Our team's pedigree allowed us to engineer sophisticated algorithms that synthesized the vast amounts of data, extracting patterns and insights not discernible through traditional methods.
Weather Data Integration: We obtained county-level data on precipitation, high-low-mean temperatures, growing degree days, and the palmer index. This data was instrumental in developing an index of "adverse weather events" for each county.
Feature Engineering: Our expertise in feature engineering allowed us to identify and craft meaningful input variables from the raw data, enhancing the predictive power of our models. By understanding the intricate relationships between various data points, we could create features that captured the essence of the impact on grain yields.
Machine Learning Model Development and Depth: Utilizing multiple proprietary machine learning models, including Random Forest, Gradient Boosting, and Neural Network models, we evaluated the historical impact of "adverse weather events" on production data over a 15-year span. Our deep understanding of these models enabled us to extract maximum predictive power from the data.
Parameter Tuning: Our team meticulously tuned the parameters of each model, ensuring optimal performance. This iterative process involved adjusting various hyperparameters to achieve the best balance between bias and variance, ensuring accurate and generalizable predictions.
Integration with Client Expertise: By combining the co-operative's domain knowledge with our data mining, architecture, analysis, and machine learning approach, we empowered their senior management team. They were better equipped to evaluate alternative strategies, including optimizing hedging positions, aligning logistics, and tailoring insurance strategies based on refined grain yield forecasts.
Outcome:
The implementation of the machine learning model, bolstered by our unique data collection methods, transformed the co-operative's approach to grain yield forecasting. With more accurate predictions, the co-operative could make informed decisions regarding hedging, optimize logistics like rail and freight, and align insurance strategies more effectively. This not only enhanced operational efficiency but also provided its farmer members with more reliable support and services.
Key Takeaways:
Accurate grain yield forecasts are crucial for making informed decisions regarding hedging, logistics, and insurance.
Deep expertise in feature engineering and parameter tuning is essential for developing robust machine learning models.
Combining domain expertise with machine learning insights empowers businesses to optimize operations and provide better services to members.
Agricultural co-operatives can harness the power of machine learning to refine their forecasts, leading to optimized operations and better support for their members.
This case study emphasizes the transformative power of machine learning in the agricultural sector, especially when combined with proprietary data collection methods. It showcases how co-operatives can make data-driven decisions that optimize operations and benefit their members, leveraging the unique expertise and pedigree of specialized teams.