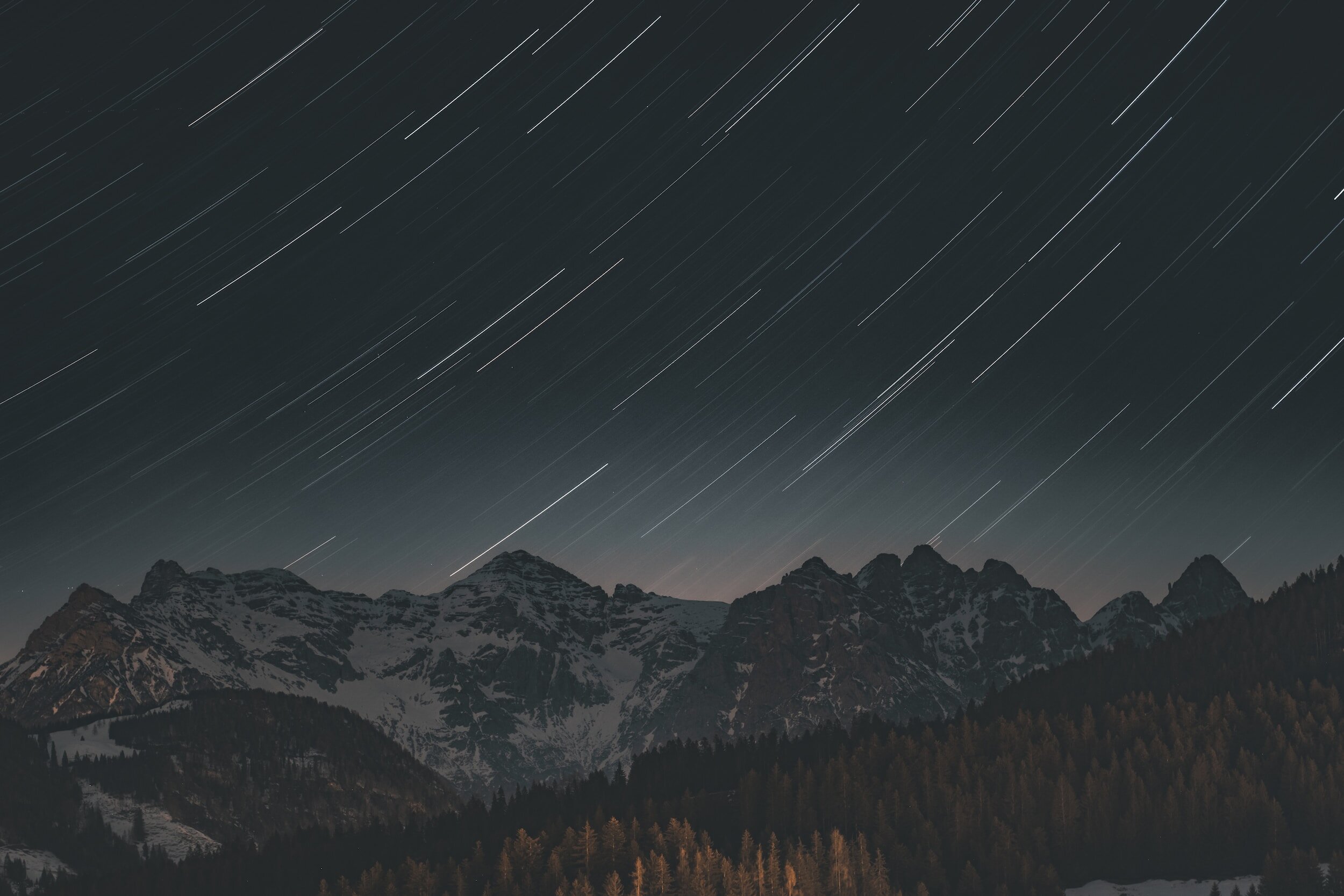
Transform Your Strategy:
See How Scoring & Segmentation Shape Success.
Scoring & Segmentation
Client Background:
A prominent player in the third-party collection industry, the client faced challenges in optimizing resource allocation to accelerate cash flow in their collection practice.
Objectives:
Better utilize finite resources to enhance collection efficiency.
Accelerate cash flow by targeting high-propensity payers.
Implement a machine learning-based payer propensity model.
Gain insights into factors affecting payment likelihood.
Challenge:
The client sought a method to predict the likelihood of a delinquent consumer making a payment before expending resources in the collection process.
Approach:
Data Preparation: Our team initiated a comprehensive analysis of the client's historical data, including account information, payment history, and demographic data. The data was cleaned and preprocessed to remove any missing values or outliers.
Feature Engineering: Key data points that could influence payment likelihood were identified. These features were then engineered to be used in the machine learning model.
Model Selection and Training: Based on the nature of the problem, a suitable machine learning algorithm was chosen. The preprocessed data was used to train this algorithm, creating a binomial classifier.
Model Evaluation: The developed model was rigorously evaluated using various metrics such as accuracy, precision, recall, and F1-score to ensure it met the client's requirements.
Deployment and Dynamic Scoring: The final model, with a true positive rate of 88%, was deployed. This meant it could successfully predict 88 out of every 100 real payers before any collection efforts were initiated. The model also produced a dynamic probability score that adjusted based on ongoing interactions between the organization and the consumer.
Outcome:
The implementation of the machine learning-based payer propensity model revolutionized the client's operations. With the ability to prioritize collection efforts based on payment likelihood, the client achieved cost savings of $1.7 million annually and witnessed a revenue growth of $4.7 million. This strategic shift in operations led to increased efficiency and profitability. Moreover, the insights derived from the model provided a deeper understanding of factors influencing payment likelihood, offering avenues for further optimization in the future.
Key Takeaways:
Machine learning can significantly enhance efficiency in the third-party collection industry by predicting payer propensity.
Proper data preparation and feature engineering are crucial for the success of such models.
Dynamic scoring models can adapt based on ongoing interactions, providing real-time insights.
Implementing such models can lead to substantial cost savings and revenue growth, transforming operations and strategy.
This case study underscores the transformative power of machine learning in the third-party collection industry, enabling businesses to make data-driven decisions and optimize resource allocation.